Your AI-native startup ain’t the same as a typical SaaS company
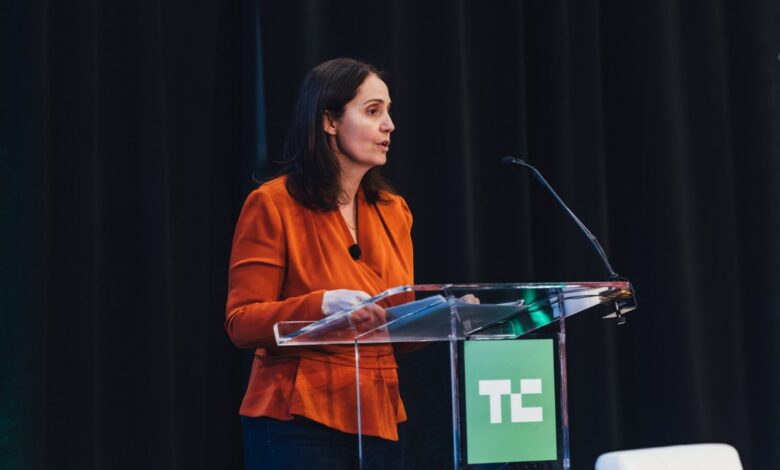
AI startups face a different set of challenges from your typical SaaS company. That was the message from Rudina Seseri, founder and managing partner at Glasswing Ventures, last week at the TechCrunch Early Stage event in Boston.
Seseri made it clear that just because you connect to some AI APIs, it doesn’t make you an AI company. “And by AI-native I don’t mean you’re slapping a shiny wrapper with some call to OpenAI or Anthropic with a user interface that’s human-like and you’re an AI company,” Seseri said. “I mean when you truly have algorithms and data at the core and part of the value creation that you are delivering.”
Seseri says that means that there are major differences in how customers and investors judge an AI company versus a SaaS startup, and it’s important to understand the differences. For starters, you can put something that’s far from finished into the world with SaaS. You can’t do that with AI for a variety of reasons.
“Here’s the thing: With the SaaS product you code, you QA and you kind of get the beta — it’s not the finished product, but you can get it out there and can get going,” she said.
AI is a completely different animal: You can’t just put something out there and hope for the best. That’s because an AI product requires time for the model to get to a point where it is mature enough to work for actual customers and for them to trust it in a business context.
“In the early days, it’s a steep curve in learning and training the algorithm, and yet it has to be good enough for the customer to want to buy so it has to be good enough for you to create value,” she said. And that’s a hard line to find for an early stage startup.
And this makes it more challenging to find early adopters. She says you want to avoid the long call where the buyer is just trying to learn about AI. Startup founders don’t have time for calls like that. She says it’s important to focus on your product and help the buyer understand your value proposition, even if it’s not quite there yet.
“Always articulate the problem you are solving and what metric — how are you measuring it?” she said. Optimize on what matters to the buyer. “So you’re solving a problem that has business decision outcomes.” It’s OK to articulate your vision, but always be grounding your discussion in business priorities and how those are informing your algorithms.
How can AI startups win?
As you build your business, you need to be thinking about how you can stake a defensible place in AI, something that is particularly challenging as the big players continually carve out huge chunks of business ideas.
Seseri points out that in the cloud era, we had a foundation layer where the infrastructure players staked their claim; a middle layer where the platform players lived; and at the top we have the application layer where SaaS lived.
With the cloud, a few players like Amazon, Microsoft and Google emerged to control infrastructure. The foundation layer in AI is where the large language models live, and a few players like OpenAI and Anthropic have emerged. While you could argue these are startups, they aren’t in the true sense because they are being financed by those same big players who dominate the infrastructure market.
“If you’re going to compete for a new foundation layer, or you know, LLM play, it’s going to be very tough with multibillion dollar capital requirements, and at the end of the day, chances are it will end up being a commodity,” she said.
At the top of the stack is the application layer where thousands of SaaS companies were able to take advantage of in the cloud era. She said that the big players like Amazon, Google and Microsoft were not able to take all of the application layer business and there was room for startups to develop and grow into large, successful businesses.
There is also a middle layer where the plumbing gets done. She points to companies like Snowflake that have succeeded in building successful businesses in the middle layer by providing a place for the application players to put their data.
So where is she investing when it comes to AI? “I put my dollars in the application layer and very selectively in the middle layer. Because I think there is a moat around algorithms, whether it’s algorithms that are proprietary to you, or open source — and data. You don’t need to own the data. But if I have to pick, I’d like to have unique data access and unique algorithms. If I am forced to pick one, I will go after data,” she said.
Building an AI startup surely isn’t easy, perhaps even more challenging than a SaaS startup. But it’s where the future is, and companies that are going to try it have to know what they’re up against and build accordingly.
Source link